Scout InsurTech Spotlight with Michael Reznik
- Michael Fiedel
- Feb 25
- 4 min read
Michael Reznik is the Head of Data + Analytics at The Baldwin Group (BWIN), an award-winning, entrepreneur-led advisory firm delivering insurance, risk mitigation, employee benefits and wealth management solutions for businesses and individuals. Michael was interviewed by Anthony Habayeb, CEO and Co-Founder at Monitaur.
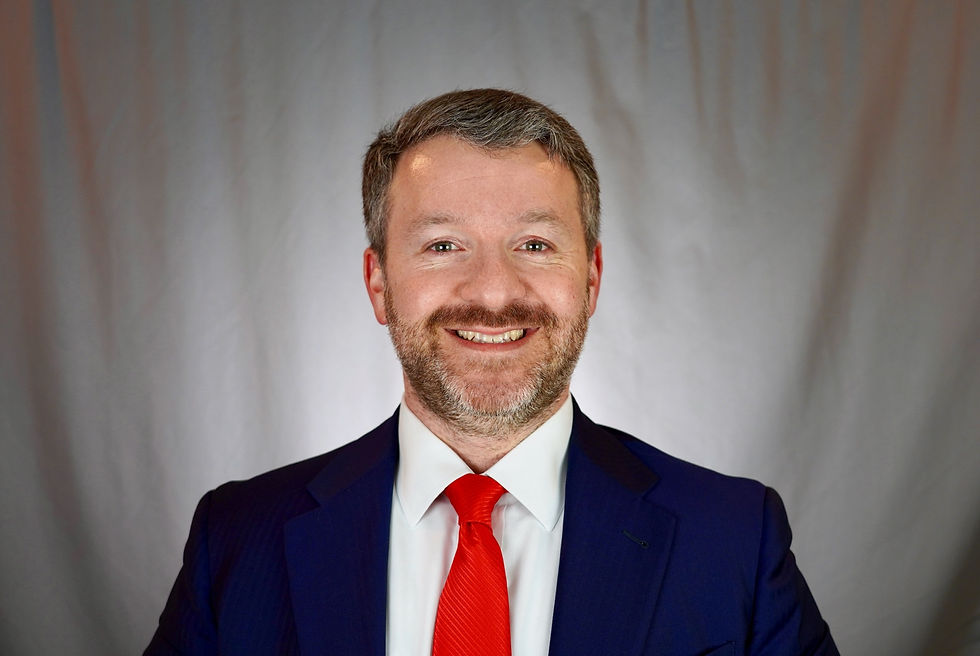
Mike, what's the brokerage opportunity in data analytics and AI? How’s it distinct from a carrier's approach?
“Generally, carriers today focus heavily on loss ratio and combined ratio. Their advanced analytics center on underwriting guidelines, product pricing, segmentation and strategies to minimize losses while ensuring pricing aligns with expected payouts.
As a brokerage, we certainly care about losses, but we aren’t the ones paying out claims. There’s no balance sheet exposure for us. Our focus is on advising clients on risk and risk mitigation, placing risk with the right carriers and empowering our advisor to be more productive and effective in serving our clients.
We approach analytics, data science, AI and machine learning with different goals than a carrier. We use analytics to score leads, determine the best carrier placement and assign the right advisors or service teams to a request. We also aim to reduce third-party data calls for placing risks—we don’t want to quote everything indiscriminately. Instead, we want to consolidate multiple quotes into meaningful insights for our customers, showing them how we’ve assessed several carriers and providing strategic advice on risk mitigation.”
Generative AI has become an almost singular AI strategic focus for many, what are your thoughts about how people should consider use of GenAI?
“The challenge in the industry right now is that we often see a solution looking for a problem. There's a lot of pressure to embrace generative AI, but when you examine real-world applications with tangible business benefits, the use cases are far more limited than people assume.
Often, the ROI of improving traditional machine learning and deep learning capabilities far outweighs generative AI initiatives. Generative AI has its place, but the key question is whether we’re over-indexing on it. In many cases, an intelligent automation or a well-designed agent is a more practical solution.
People are also realizing that generative AI requires significant maintenance—models evolve, experience drift and must be updated, validated and governed. Just because a company can build a chatbot trained on internal data doesn’t mean it will drive meaningful ROI. On the other hand, a predictive pricing or risk model embedded across all customer interactions can deliver substantial returns much faster.
That’s not to say traditional AI doesn’t require monitoring, but its governance tends to be more mathematical, scientific and cost-effective compared to generative AI. Right now, I think the industry has over-invested in generative AI without fully leveraging traditional ML and data science techniques.”
Do you have any advice for brokers—or analytics leaders in general—on fostering a culture that promotes the right decision-making?
“The key is structured innovation. Eric Ries’ The Lean Startup lays out a great approach to handling innovation in a measured way.
We don’t discourage exploration of generative AI, but we establish clear criteria for success. What problem are we solving? How will we measure ROI? When do we pivot?
At my company, we encourage experimentation in a sandbox environment where teams can explore and build capabilities. However, we focus on creating scalable, repeatable solutions that can be applied across multiple use cases.
For example, as a brokerage, we handle a lot of unstructured data. Initially, we evaluated vendors for document intelligence solutions. But, generative AI tools evolved quickly, and existing platforms like AWS Bedrock and Microsoft’s AI Studio matured. So, instead of continuing to pay vendors, we built our own document extraction framework that supports multiple use cases—processing declaration pages, loss runs and policy docs.
To prevent over-indexing on generative AI, we apply traditional project management principles: cost-benefit analysis, success metrics and scalability assessments. Our approach ensures that when we invest, we maximize impact across the organization.”
Are there critical infrastructure elements that help unlock AI’s potential while mitigating concerns about overuse or misalignment?
“The old principle of ‘garbage in, garbage out’ applies—models are only as good as the data fed into them.
We’re not building our own large language models (LLMs); we leverage existing ones. The key question is: What data do we expose, and how do we govern it? This applies to both generative AI and traditional ML models.
From an infrastructure perspective, we focus on ML Ops and AI governance. We control data access, track model inputs and outputs and manage data lifecycles. We create sandbox environments with structured access controls.
For example, in our AWS Databricks environments, we are using MLflow to manage models, and we tightly regulate data ingress and egress. This allows us to innovate while ensuring security, governance and scalability.”
How do you decide what to buy versus build—both for operational enablement and end-user applications?
“It’s an evolving balance. Initially, we purchased generative AI services, but they were expensive—costing per page or per token. As vendor tools matured, Microsoft’s Azure Open AI models and AI Studio provided low-code options that allowed us to build similar capabilities in-house.
Now, if we build, we focus on scalability—ensuring we can reuse solutions across multiple use cases. But some services are still more cost-effective to buy, especially those involving proprietary third-party data.
A key factor is capacity. If a business unit has an immediate need and lacks bandwidth, we support them in purchasing a solution. Later, we evaluate whether to bring it in-house.
For example, we initially used third-party lead scoring tools. Over time, we built our own ML models based on insights from those tools, adding features the vendors didn’t provide.”
Looking a year ahead, where do you see Baldwin in its AI strategy?
“A year isn’t long, but we’re focused on building scalable, reusable AI services to support profitable growth. A major priority is improving data governance and literacy.
Technology alone won’t drive change—our organization needs to understand and effectively use data products. So, our biggest focus will be increasing data literacy, ensuring that everyone in the company becomes a steward of the information they handle. That’s how we’ll drive adoption and long-term success.”